Measuring Impact: KPI's and Metrics for Industry-Wide Skills Interventions
This Duja Consulting paper examines how to accurately assess the true value of industry-wide skills interventions – and why it matters more than ever.
As businesses, policymakers, and educators continue to invest in skills development on a large scale, one critical question remains: How do we know if it’s working?
Our latest paper, “Measuring Impact: KPIs and Metrics for Industry-Wide Skills Interventions,” provides practical, business-focused insights into evaluating these efforts using meaningful data.
Here’s what you’ll find in the paper:
- Why Measuring Impact Matters – Especially When Aligning Skills Initiatives with Broader Business and Economic Outcomes.
- Key frameworks to evaluate interventions, including logic models, Balanced Scorecards, and Kirkpatrick’s levels.
- Essential metrics and KPIs – from individual skills attainment to organisational productivity and sector-wide growth.
- Real-world case study from the manufacturing sector that demonstrates how targeted upskilling drove measurable results.
- Common challenges in data collection, evaluation, and attribution – and how to overcome them.
- Clear recommendations for businesses, policymakers, and training providers to strengthen impact measurement.
- If you’re investing in workforce development and want to ensure your interventions drive measurable change, this paper is a must-read.
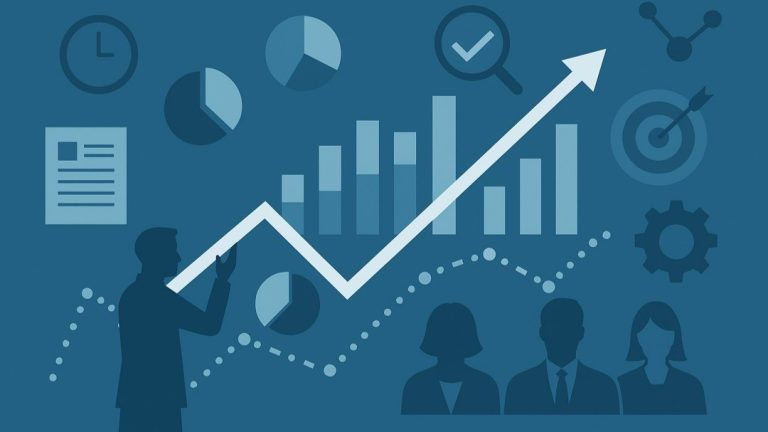
Introduction
Industry-wide skills interventions are large-scale initiatives aimed at improving the capabilities of the workforce across an entire sector or region. These can include government-funded training programmes, cross-company upskilling efforts led by industry associations, or educational reforms aligned to industry needs. In today’s fast-changing economy, skill gaps pose a significant challenge – in fact, an inability to find skilled talent is cited as one of the biggest barriers to business growth and industry transformation across many sectors. To address such gaps, businesses, policymakers and educational institutions invest heavily in developing critical skills at scale. However, ensuring these investments deliver real value requires robust methods to measure their impact. This report examines how to assess the outcomes of industry-wide skills interventions, discussing key performance indicators (KPIs), relevant evaluation frameworks (such as logic models and the Balanced Scorecard), and practical approaches to evaluate success at scale. A case study is included to illustrate these concepts in action. The focus is business-oriented, providing insight for companies, policymakers, and educators on why measuring impact matters and how to do it effectively.
Importance of Measuring Impact
Investing in workforce skills is costly and time-consuming, so stakeholders need evidence that these interventions are working. What gets measured gets managed – by tracking the right metrics, organisations can ensure training initiatives stay aligned with business and policy goals and make adjustments for improvement. Measuring impact also helps demonstrate return on investment (ROI) to senior leadership and funders. For example, one multinational (IBM) implemented a rigorous competency evaluation programme and saw a 20% improvement in employee performance metrics over three years, along with a 15% reduction in staff turnover. This outcome showcases how a structured approach to assessing and developing skills can drive tangible business results. In general, linking skills programmes to broader goals like productivity, quality, or innovation is vital. A recent report emphasises that organisations need to establish clear criteria to measure and document the benefits, ROI and impact of skills initiatives, tracking progress on metrics such as financial outcomes (e.g. revenue growth), innovation (e.g. new products launched), and people metrics (e.g. employee engagement). Without such measurement, companies may not know if an industry-wide training effort actually improved performance, and policymakers would lack the data to justify continued funding or to scale successful models. In short, measuring impact provides accountability and learning: it proves whether the intervention delivers value and informs how future skills programmes can be more effective.
Frameworks for Evaluating Skills Interventions
When assessing large-scale skills interventions, it helps to use established evaluation frameworks to structure the measurement of outcomes. Two useful approaches are logic models and the Balanced Scorecard, alongside training-specific models like Kirkpatrick’s four levels.
- Logic Model: A logic model lays out the chain from inputs and activities to outputs, outcomes, and long-term impact. This framework distinguishes what a programme directly produces versus the broader results it influences. For a skills intervention, inputs might be funding and training hours, outputs could be number of workers trained or certifications earned, and outcomes would include changes in workplace behaviour or productivity gains. Ultimately, the impact could be seen in industry-wide indicators like improved productivity or reduced skills shortages. Using a logic model helps evaluators ensure they capture both immediate outputs (e.g. people trained) and ultimate outcomes (e.g. business performance improvements). It also clarifies that while we control outputs, higher-level outcomes are often influenced by other factors, underlining the importance of careful attribution.
- Balanced Scorecard (BSC): Originally a strategic management tool, the Balanced Scorecard can be adapted to skills programmes to ensure a balanced set of metrics. The BSC advocates measuring performance from four perspectives: learning and growth, internal processes, customer (or stakeholder), and financial. Applying this to a skills initiative, learning and growth metrics might track skill acquisition and employee development (e.g. training hours, skill assessment scores); internal process metrics could gauge how effectively the training is delivered and applied on the job; customer or stakeholder metrics might measure effects on customer satisfaction or employer satisfaction with workforce skills; and financial metrics would look at ROI, such as increased revenue or cost savings attributable to the upskilling. The Balanced Scorecard approach explicitly links learning interventions to business outcomes – for instance, improving employees’ skills should enhance internal processes, which in turn boosts customer outcomes and financial results. This top-down linkage helps organisations verify that a skills programme contributes to strategic goals, not just to vague “learning” in isolation.
- Kirkpatrick’s Four Levels: Another widely used framework (particularly in training evaluation) is Donald Kirkpatrick’s model, which measures training effectiveness at four levels: Reaction (how participants felt about the training), Learning (knowledge or skills gained), Behaviour (changes in on-the-job behaviour or performance), and Results (the impact on organisational outcomes). Kirkpatrick’s framework stresses moving beyond participant satisfaction to whether the training translated into workplace improvements and business results. Level 4 (Results) is especially pertinent for industry-wide interventions, as it asks whether the programme achieved key business outcomes – for example, higher productivity, fewer accidents, increased sales, or other tangible results. Using this model, evaluators of a sector-wide skills programme would collect data not only on how trainees rated the course or improved their test scores, but also on metrics like productivity gains, quality improvements, or financial returns observed after implementation. Kirkpatrick’s approach, like the others, reinforces the principle that measuring impact means looking at real-world results, not just training inputs.
In practice, these frameworks are complementary. A logic model provides a roadmap of how an intervention is supposed to lead to impact, the Balanced Scorecard ensures a holistic view of performance indicators, and Kirkpatrick’s levels offer a stepwise check on whether learning translated into desired outcomes. Together, they help stakeholders design measurement plans that capture both the micro-level effects (e.g. skills gained by individuals) and the macro-level outcomes (e.g. organisational or industry improvements).
Types of KPIs and Metrics Used
When evaluating skills interventions at scale, it’s important to choose KPIs that collectively paint a picture of both the implementation and the impact of the programme. Below are the main types of metrics used, ranging from immediate measures of activity to long-term indicators of success:
- Participation and Engagement Metrics: These gauge the reach and uptake of the programme. For example, enrolment numbers and attendance rates show how many people the intervention engaged and how consistently they participated. Completion rates indicate the proportion of participants who finished the training, reflecting the programme’s ability to keep learners on track. High participation and completion rates suggest the intervention is accessible and relevant, while drop-off rates might highlight issues with content or delivery. Stakeholders also often look at participant feedback or satisfaction surveys (sometimes called “reaction” measures) – positive feedback can signal that the training was engaging and useful, which is a good first step toward impact.
- Skill Attainment Metrics: These metrics assess whether the intervention actually improved the skills or knowledge of participants. Common measures include pre- and post-training assessment scores to quantify learning gain, certification or qualification attainment rates (if the programme includes credentials), and practical skill demonstrations or simulations. For instance, an IT skills programme might track how many participants earned an industry certification or improved their proficiency test scores. These are outcomes at the individual level – they demonstrate that the workforce’s skill levels have increased as a result of the intervention. In some cases, digital badging or competency frameworks are used to measure skills acquisition across an industry in a consistent way.
- Application and Behaviour Change Metrics: A critical question is whether newly acquired skills are being applied on the job. Metrics here might include on-the-job performance indicators or behavioural assessments after the training. For example, companies can track if there was a reduction in error rates, faster project delivery times, or improved quality control following a skills programme. If the intervention focused on soft skills (e.g. leadership or customer service), one could measure changes in employee behaviour through 360-degree feedback or supervisor evaluations. In training parlance, this aligns with Kirkpatrick’s Level 3 (Behaviour), examining whether employees are utilising their new skills in practice. An example would be tracking a safety training’s effect by monitoring the number of safety incidents before and after across an entire industry. Improved performance metrics in the workplace signal that the skills intervention has translated from the classroom to the job.
- Organisational and Industry Outcomes: These are higher-level KPIs that reflect the broader impact on the organisation or sector. Businesses often look at outcomes like productivity gains, quality improvements, innovation, and customer satisfaction as indicators of success. For instance, a company might measure whether a digital skills upskilling programme led to more new products or services being developed (an innovation metric) or higher customer satisfaction scores due to better service quality. Similarly, employee retention is a valuable metric – effective development programmes can boost morale and loyalty, reducing turnover rates. (Notably, one study found companies with strong learning and development programmes experience 52% lower employee turnover on average.) At an industry or economy-wide level, policymakers might track metrics such as employment rates of programme graduates, wage growth for those who underwent training, or reductions in the number of job vacancies in high-skill roles as a result of increasing the talent pool. These metrics connect the skills intervention to economic and social outcomes like improved employability and income. A workforce development programme, for example, may report a job placement rate (percentage of trainees who secure employment) or an average wage increase for participants – both being direct indicators of economic impact.
- Return on Investment and Cost-Benefit Metrics: Ultimately, many stakeholders will ask: did the benefits outweigh the costs? ROI analysis is a more advanced metric that compares the monetary value of the outcomes (e.g. increased productivity, higher revenues, cost savings) to the cost of the skills programme. Calculating ROI can be complex but powerful – for instance, an industry association might demonstrate that a training initiative costing £1M yielded an estimated £3M in productivity improvements across member companies, equating to a 3:1 return. Other related measures include cost per participant or cost per successful outcome, and any savings gained (such as reducing the need for external hiring by upskilling internal staff, which saves recruitment costs). While financial metrics should not be the sole focus, they provide a clear language to justify interventions in business terms. As one training executive noted, being able to relate learning outcomes to profitability and revenue helps answer the CEO’s question about ROI of training.
It’s important to use a mix of leading and lagging indicators among these KPIs. Leading indicators (like training completion or knowledge test scores) signal progress early on, while lagging indicators (like annual productivity or retention rates) show ultimate impact but take time to observe. By combining multiple types of metrics, evaluators can build a holistic view. For example, a skills programme’s success might be evidenced by high participation and certification rates (leading indicators) and, a year later, by measurable improvements in productivity and quality for the firms involved (lagging indicators). Indeed, experts recommend using both quantitative measures (e.g. numbers and percentages) and qualitative feedback (e.g. testimonials, case narratives) to capture the full scope of impact. This ensures that the metrics are not just about counting outputs but also about understanding outcomes in context.
Case Study: Upskilling in the Manufacturing Sector
Case Study – Ford Motor Company: To illustrate these concepts, consider a skills intervention in the automotive manufacturing industry. Ford Motor Company faced a challenge in the 2010s: rapid technological advancements (automation, smart technologies) were outpacing the skills of their existing workforce. In response, Ford launched a comprehensive upskilling and competency evaluation programme (internally referred to as the “Ford Smart Mobility” initiative) to prepare employees for new manufacturing processes and digital tools. The programme began by assessing the current skill levels of thousands of Ford’s production staff and identifying gaps relative to the skills needed for future roles (for example, skills in operating advanced robotics and data-driven decision-making on the factory floor). Using this data, Ford tailored training modules for different groups of employees, ranging from technical courses on new machinery to coaching in problem-solving and teamwork.
As Ford implemented the training, it also established clear KPIs to track progress. These included the percentage of workers certified on new equipment, the error rate in production, and throughput time on certain assembly tasks. Crucially, Ford measured before-and-after performance for both individuals and production teams. Within a year of rolling out the programme, the company began to see significant improvements. By leveraging metrics from the initial skills assessment and the follow-up performance tracking, Ford reported a 30% increase in overall productivity on the manufacturing line, alongside notable gains in employee satisfaction. In other words, plants were able to produce more output per worker, and surveys showed workers felt more confident and valued in their upgraded roles. This 30% productivity jump is substantial in an industry typically focused on incremental efficiency gains – it showcased the powerful impact of aligning workforce skills with evolving organisational goals. Ford’s management also observed secondary benefits: improved quality (defect rates dropped in the lines where employees were upskilled) and reduced need to hire external specialists because internal staff could fill advanced technical roles. The programme’s success was communicated across the automotive sector, and it became a reference point for industry-wide upskilling efforts.
Key takeaway: The Ford case study highlights how a well-planned skills intervention, supported by a framework of competency assessment and targeted training, can be measured in terms of concrete business outcomes. By setting up the right metrics (from individual skill acquisition to team productivity) and monitoring them throughout the intervention, stakeholders could demonstrate value. This example, though within one company, had industry-wide resonance – it showed other manufacturers that investing in upskilling could yield measurable returns in productivity, quality, and workforce morale. It underlines that effective impact measurement not only proves results but can also build the case for scaling such interventions across an entire sector.
Challenges in Data Collection and Evaluation
Measuring the impact of skills interventions at scale is not without challenges. Stakeholders often encounter several difficulties in gathering reliable data and evaluating outcomes:
- Data Fragmentation and Silos: Industry-wide programmes involve multiple organisations (companies, training providers, government agencies), and data about participants and outcomes may be spread across different systems. It can be hard to compile a complete picture when, for example, training providers hold data on course completion, employers hold data on job performance, and government holds data on employment or wages. A lack of integration means evaluators struggle to connect the dots. In some cases, important data is simply not available or not shared – one report noted that local labour market data and workforce outcome data are often not aligned or synchronised between agencies and businesses. Ensuring data compatibility and sharing across institutions is a major logistical hurdle.
- Attribution of Outcomes: When positive changes are observed (like a productivity increase or lower unemployment in a sector), attributing these results directly to the skills intervention is challenging. Many factors can influence outcomes in parallel – economic conditions, technological changes, or other initiatives running concurrently. The logic model reminds us that we control outputs but only influence outcomes. Thus, evaluators must use careful methods (control groups, baseline comparisons, longitudinal tracking) to isolate the effect of the training programme. Without such rigour, there’s a risk of over-claiming (taking credit for effects the training didn’t actually cause) or under-claiming (failing to recognise real benefits because they are obscured by other variables).
- Inconsistent KPIs and Definitions: Different stakeholders might track impact differently, leading to inconsistency. One company might define “job placement” one way while another defines it differently, or educational institutions might measure skill proficiency using different tests. This makes industry-wide analysis difficult – it’s comparing apples to oranges. Developing common metrics or standards (for example, a standard definition of what counts as a “skilled worker” in an industry, or a common skills taxonomy) can mitigate this, but achieving agreement is not easy. Without standardisation, data collection can yield results that are not directly comparable or aggregable.
- Data Quality and Collection Burden: Collecting impact data often relies on self-reports, surveys or administrative follow-up, which can suffer from low response rates and biases. If employees fill out a survey on how they applied new skills, some may not respond or may give overly positive answers. Similarly, tracking long-term outcomes (like career advancement or income changes years after training) can require linking to government records or doing extensive follow-up surveys, which is costly and time-intensive. Ensuring data quality (accuracy, completeness, timeliness) is a continual challenge. A comprehensive plan is needed to gather data – including setting a baseline before the intervention and using consistent methods – but not all programmes have the resources or expertise to do this well.
- Privacy and Legal Concerns: Sharing data on individuals between companies, educators and government can raise privacy issues. Regulations (such as data protection laws) may limit how participant information is shared or used, especially if it involves sensitive personal data. Navigating these legal requirements (e.g. obtaining consent, anonymising data) is necessary to collect and combine data from multiple sources. In some cases, laws that restrict data sharing between education and labour departments can impede the creation of unified longitudinal datasets. Policymakers often need to find ways to enable data exchange while respecting privacy – not a trivial challenge.
- Measuring Intangibles and Long-Term Impact: Some benefits of skills interventions are qualitative or emerge in the long term. For example, improved teamwork, creativity, or adaptability in the workforce are desired outcomes but are hard to measure directly. Likewise, the full economic impact of a major upskilling initiative might play out over many years as companies innovate and workers advance in their careers. Capturing these intangibles may require proxy indicators or qualitative research (interviews, case studies) to supplement hard metrics. There is also the issue of time lag – stakeholders may want to see results within a year, but the true impact (say, on innovation or on reducing societal skills gaps) could take several years to manifest, well beyond typical reporting cycles.
In summary, while it is essential to measure impact, one must be aware of these evaluation challenges. Overcoming them involves careful planning of data collection, choosing the right indicators, and often, collaborating across organisations to share data and insights. It also requires transparency about limitations – recognising, for instance, when data is incomplete or when results can only suggest correlations rather than proven causation.
Recommendations for Stakeholders
To improve the measurement of skills interventions and ensure stakeholders get actionable insights, the following recommendations are offered for key groups involved:
- For Businesses: Companies should align skills intervention metrics with their strategic business goals. This means identifying KPIs that matter to organisational success (e.g. project delivery time, customer satisfaction, revenue per employee) and tracking how training influences those indicators. Adopt tools like the Balanced Scorecard to integrate learning metrics into regular performance management. It’s also recommended to establish a baseline before training – for example, measure current productivity or error rates – so that post-training improvements can be quantified. Businesses can invest in technology (learning management systems, analytics platforms) to collect data on employee learning and performance seamlessly. Furthermore, creating a culture of measurement is key: encourage managers and employees to treat training not as a tick-box exercise but as something whose impact will be reviewed and discussed. This might involve setting up regular review meetings where training outcomes are presented alongside financial results, reinforcing that leadership cares about ROI on skills development. Finally, businesses should be willing to share anonymised data and best practices through industry forums, so that benchmarking is possible across the sector.
- For Policymakers and Industry Bodies: Government agencies and industry associations play a crucial coordinating role, especially for sector-wide initiatives. Policymakers should push for common evaluation frameworks and standards. For instance, they can develop industry-wide competency frameworks and standardised metrics (perhaps a common “skills score” or certification standards) so that outcomes from different programmes can be compared. Investing in data infrastructure is critical – consider creating or supporting a centralised platform or data trust where outcomes (employment, earnings, etc.) of training participants can be tracked longitudinally (with privacy safeguards). In the UK context, for example, linking education and tax records has allowed analysis of learners’ earnings after apprenticeships; such data integration efforts should be expanded. Policymakers should also require or incentivise robust evaluation in funding agreements – e.g. mandating that any government-funded skills programme uses a logic model and reports against predefined KPIs. Ensuring that programmes set SMART objectives and targets at the outset (Specific, Measurable, Achievable, Relevant, Time-bound goals) will make later impact measurement more straightforward. Additionally, governments can facilitate partnerships between employers and educational institutions to share outcome data, and they might commission independent impact studies (using techniques like control groups or econometric analysis) to get an unbiased view of what works. On a practical level, simplifying data-sharing regulations or providing clear guidance can remove barriers to collaboration – for example, clarifying how training providers can share participant outcomes with researchers in compliance with data protection laws. The aim for policymakers should be to create an ecosystem where measuring impact is standard practice and supported by infrastructure, rather than an afterthought.
- For Educational Institutions and Training Providers: Colleges, universities, and private training providers delivering the skills programmes should embed evaluation into programme design from the start. This includes defining outcome metrics for each programme (e.g. certification rate, job placement rate for graduates, employer satisfaction with graduates) and collecting the necessary data through alumni tracking and assessments. Institutions should strengthen feedback loops with employers – for example, forming advisory boards of industry partners who can report on how well graduates or trainees are performing on the job and where skill gaps remain. Such feedback is invaluable for both measuring impact and updating curriculum. Providers are encouraged to use a mixed-methods approach: quantitative data (test scores, graduation rates, etc.) plus qualitative data (surveys, interviews where graduates and employers describe the training’s effectiveness). Embracing new technologies can help; for instance, some institutions use online platforms to monitor graduates’ career progression (via LinkedIn or dedicated alumni surveys) to gauge long-term outcomes like career advancement or further learning. Education providers should also make sure to report outcomes in a transparent way. Rather than just boasting about number of people trained, reports should highlight results such as “90% of graduates found relevant employment within 6 months” or “Employers reported a 25% improvement in new hire job readiness after our programme”. This outcome-focused reporting will demonstrate value to funders and students alike. Lastly, training providers can contribute to broader impact by adopting common skill taxonomies and credential standards (in line with industry frameworks) – this makes their outcomes more comparable and meaningful at an industry-wide level, as everyone is “speaking the same language” regarding skills.
Across all stakeholder groups, some best practices stand out for effective impact measurement. First, always establish a clear baseline and goals before the intervention starts. Second, use a combination of quantitative and qualitative metrics to capture both the scale of outcomes and the stories behind them. Third, incorporate regular feedback and review – treat measurement as an ongoing process, not a one-time evaluation. Fourth, collaborate and share data responsibly: a collective effort will give a more complete picture of industry impact than isolated evaluations. By following these recommendations, stakeholders can better demonstrate the value of skills interventions and continuously improve them, ultimately fostering a more competent and competitive workforce.
Conclusion
Measuring the impact of industry-wide skills interventions is essential to ensure that efforts to upskill the workforce are genuinely delivering benefits for individuals, businesses, and the broader economy. By using structured frameworks and carefully chosen KPIs, stakeholders can move beyond anecdotes and intuition to evidence-based decision-making. The process involves challenges – from data collection hurdles to attributing results – but with planning and collaboration these can be managed. The case study from the manufacturing sector showed how a targeted upskilling programme, coupled with diligent measurement, yielded substantial productivity gains and became a model for others. The lesson for any industry is clear: defining success metrics at the start and rigorously tracking them through the life of a skills programme will pay dividends. It not only proves the value of current interventions but also illuminates how future initiatives can be sharpened. For businesses, policymakers, and educators alike, a focus on impact measurement transforms skills development from a leap of faith into a strategic, results-oriented endeavor – one that ultimately strengthens industries and communities in measurable ways.
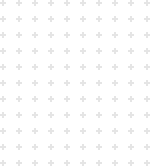
Connect with Duja Consulting today to take a fundamental step toward good governance, sustainable growth, and economic resilience.



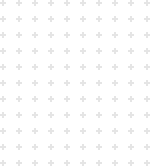
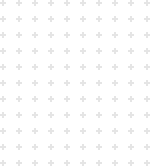
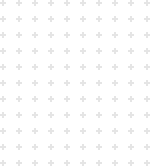


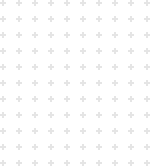
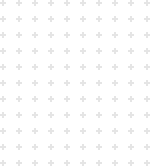
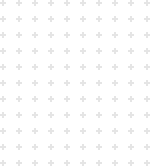
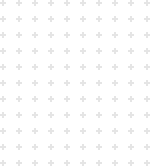
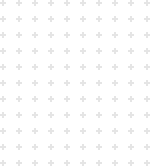


